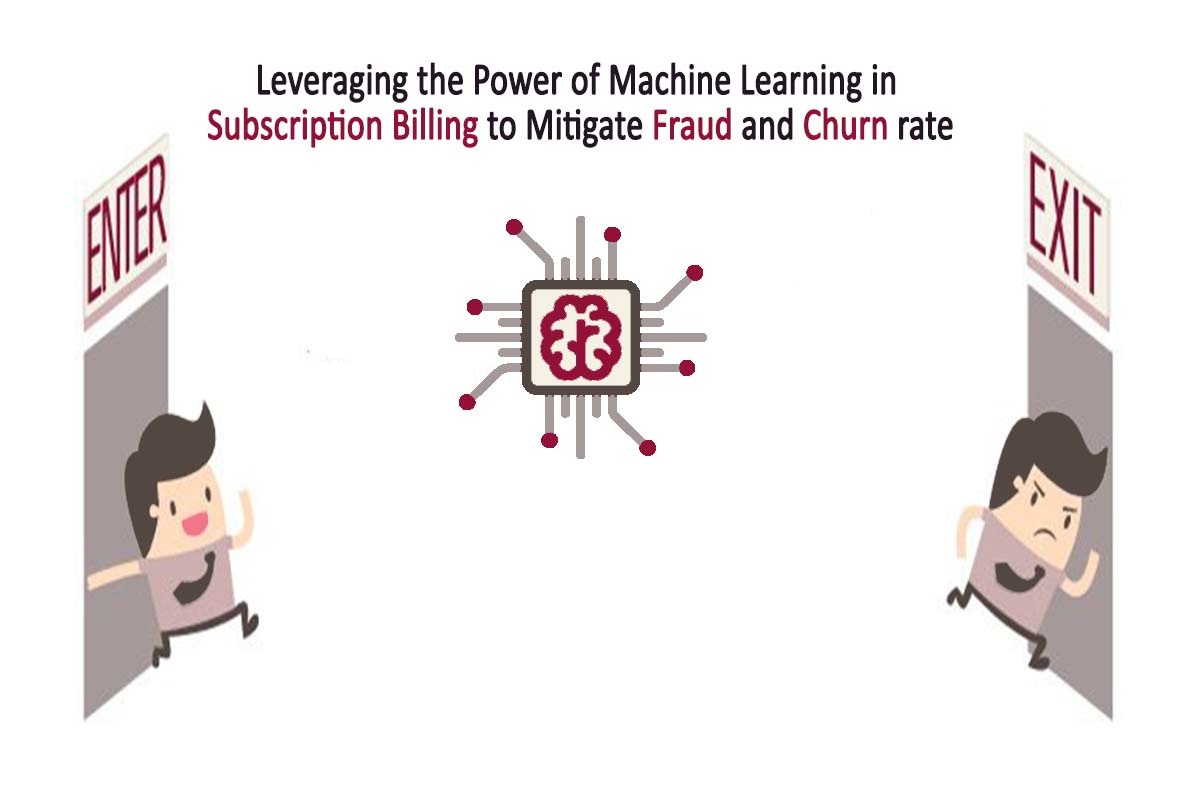
Leveraging the Power of Machine Learning in Subscription Billing to Mitigate Fraud and Churn rate
The dawn of cutting-edge technologies is grooving into the subscription-based businesses with full swing. Today, Software as a Service or SaaS enterprises are striving to meet the demands and expectations of customers by taking into consideration customer preferences. To meet the purpose, the paradigm of subscription billing is shifting towards the adoption of machine learning technology and models.
The establishment and development of machine learning models according to the use-cases of subscription billing can help automate a wide range of processes. The success metrics for businesses vary. For instance, some want to reach the ultimate goal of increased business revenue and for some, scalability and customer retention are the actual resolution.
From Manual to Automated Subscription Management
A few years back when subscription-based businesses were quite far from the prevalence of high-end technologies, the workflow used to be totally manual. Businesses used to operate with more resources, time, effort, and investments. The processes were highly prone to human errors and thus contribute to inefficiency in operational infrastructure. However, with the sharpening machine learning strong underlying algorithms and techniques, businesses are vigilant to automate their framework. This ensures the need for fewer resources, less effort, faster progress, and lesser operational cost.
Initially, a huge set of training data is required to train the machine learning model. The next step is to test the model with a huge corpus of testing data set. The model is improved with more and more data to give better predictions against the inputs and parameters. The huge corpus would consist of data related to successful payments, recurring payments data, billing failures, customer behavior, process cancellations, cart abandonment, customer lifecycle, closings, etc.
Other than this, any metadata associated with the recurring payments or customer is also included in the training data set. The machine learning model takes in the data such as the age of the customer, gender, interest, subscribed services, payment model, demographics, history as well as billing service such as music subscription, SaaS subscription, etc. and do further processing. On the huge data, the machine learning model is trained and tested on a similar but separate dataset. In this way, forecasts and predictions could be made in subscription-based businesses for better sales, revenue, and enhanced customer retention.
Machine Learning to Reduce Churn Rate
Churn has various types. It could be both voluntary churn and involuntary churn. Voluntary churn is the one in which a customer terminates the subscription on its own. Whereas, involuntary churn occurs when a subscription of the customer is terminated by the system. Usually, involuntary churn is as a result of the declined payment.
There does not exist a clear way to identify the reason why a card is declined. The customer patterns, actions, and behavior could be different towards different attributes. Each new decline could be totally different from the previous one. For instance, the subscription data, billing models, payment methods, customer lifecycle, different credit cards, payment dynamics, etc.
Manually, the identification of these patterns is quite hard. Though, machine learning models play a critical role in streamlining the predictions and estimations in a highly effective way. The identification of those parameters can be ensured through machine learning models. The data could be trained and tested over various behavioral patterns of customers. The reason behind failed payments could be dug to improve the modules which lead a customer to terminate the payment.
The factors though may not correspond to the internal system but also to the integrated payment systems which have a cumbersome payment procedure due to multiple verification’s that accelerate the process of termination. Even sometimes, the customer is unaware of the payment termination itself. This factor could lead to confusion as well as leave a bad impact on brand reputation. To curb all these outcomes, SaaS businesses have identified the potential of machine learning that help scrutinize customer behavior and allows businesses to;
- Identify the customer patterns throughout the customer lifecycle
- Identify which payment methods lead to increased churn rate
- Predict when a customer is most likely to terminate a process
- Forecast the total closings in a particular time period
- Estimate the business revenue in some time frame
- Predict when a customer would unsubscribe certain services
All these parameters individually or collectively help understand the reason behind the churn rate. Accordingly, the strategies could be reformed, processes could be remodeled and marketing campaigns could be altered. This helps increase customer retention and churn reduction to boost business revenue.
Read More: How AI-Based Subscription System Can Benefit IT Startups to Grow Revenue Empire
Machine Learning to Curb Fraud
The increasing innovations in the digital world are not only restricted to the strategies and technology adoption in businesses. Fraudsters are getting advanced enough to find out vulnerabilities and loopholes in existing systems to perform a range of malicious activities. The increasing incidents of credit card fraud and billing frauds call out for stringent mechanisms through which the payment frauds could be minimized. As a result of payment attacks, false charge-backs are another headache for SaaS businesses that affect the business revenue. Machine learning technology plays a pivotal role in combating payment frauds. With a dynamic and intelligent approach, the risks need to be mitigated in order to fight against the revolutionizing tactics of bad actors.
Machine learning algorithms in recurring billing security systems help identify the unauthorized entities trying to perform transactions. The customer behavioral patterns are identified and transactions are terminated if some unusual or suspicious activity is identified. This helps mitigate the risks of fraud and ensure a transparent and secure environment for customers and businesses to operate in. SubscriptionFlow harnesses the power of machine learning models and techniques to provide customers enhanced and optimized experience throughout the customer journey. To get a competitive edge in the market, SubscriptionFlow empowered the subscription-based SaaS business and enterprises to compete and grow at a rapid pace.